4 标准预处理[Sample Information]
方法[Method]
将Cell Ranger (v3.1.0)生成feature-barcode矩阵作为输入,通过Seurat (v3.1.1)进行一系列的质控、降维、聚类步骤。
Take Cell Ranger (v3.1.0) to generate a function-barcode matrix as an input. Seurat (v3.1.1) Perform a series of quality control, dimensionality reduction, and clustering steps .
4.1 细胞中不同特征分布[Distribution of different features in cells]
图中每个点代表的是一个细胞,反应的是对应特征在所有细胞的一个分布情况。通过下图,我们可以确定一个大概的筛选范围。以nFeature_RNA为例,可以看到数值在2000以上的细胞是非常少的,可以看做是离群值,所以在筛选时,如果一个细胞中检测到的基因个数大于2000,就可以进行过滤。
Each point in the figure represents a cell that responds to a distribution of corresponding features in all cells. By observing the above figure, we can determine an approximate screening range. Taking nFeature_RNA as an example, it can be seen that the number of cells above 2000 is very small, and can be regarded as an outlier, so if the number of genes detected in one cell is greater than 2000 at the time of screening, it can be filtered.
P
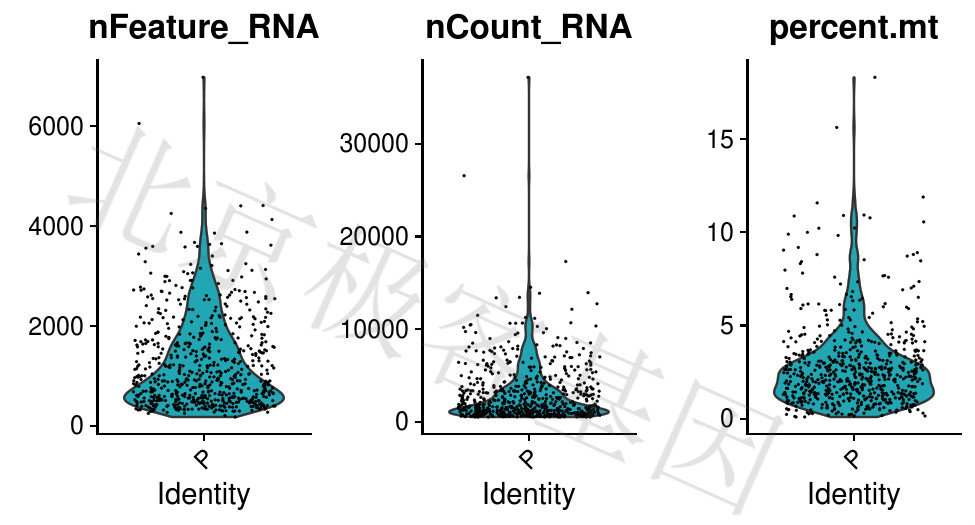
Figure 4.1: P-单个细胞中的基因数,单个细胞的表达量,线粒体基因表达量百分比
PDF 文件 : P-QC_metrics_violin.jpg
T
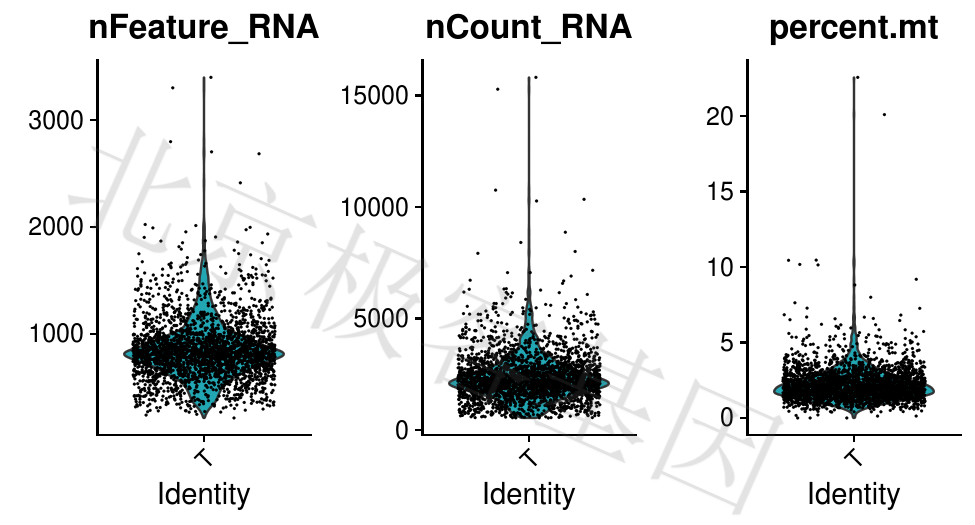
Figure 4.2: T-单个细胞中的基因数,单个细胞的表达量,线粒体基因表达量百分比
PDF 文件 : T-QC_metrics_violin.jpg
图例[Figure legends]
nFeature_RNA:该细胞中共检测到的表达量大于0的基因个数
nCount_RNA:该细胞中所有基因的表达量之和,即细胞中的所有reads Count数目
percent.mt:线粒体基因表达量的百分比,低质量或垂死细胞通常表现出较高的线粒体污染
nFeature_RNA: the total number of genes detected in this cell whose expression level is greater than 0 nCount_RNA: the sum of the expression of all genes in the cell, that is, the number of all reads Count in the cell percent.mt: the percentage of mitochondrial gene expression, low quality or dying cells usually show higher mitochondrial contamination
4.2 特征的相互关系[The relationship between features]
在过滤阈值时,我们还需要考虑一个因素,就是这3个指标之间的相互关系。
When filtering thresholds, we also need to consider a factor, which is the relationship between the three features.
P
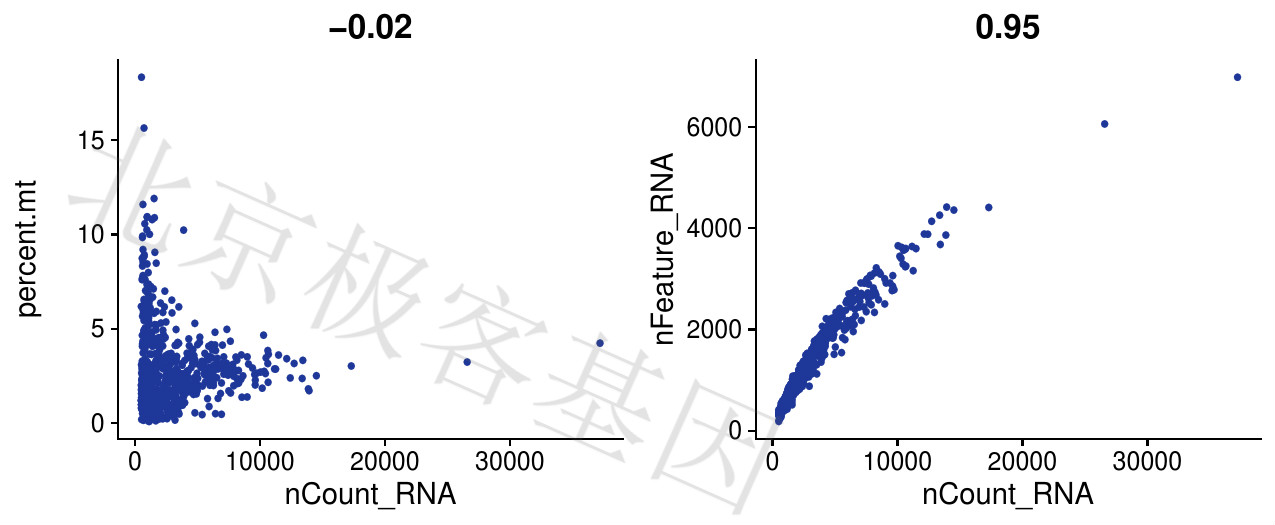
Figure 4.3: P-3个指标相互关系
PDF 文件 : P-feature_relationships.jpg
T
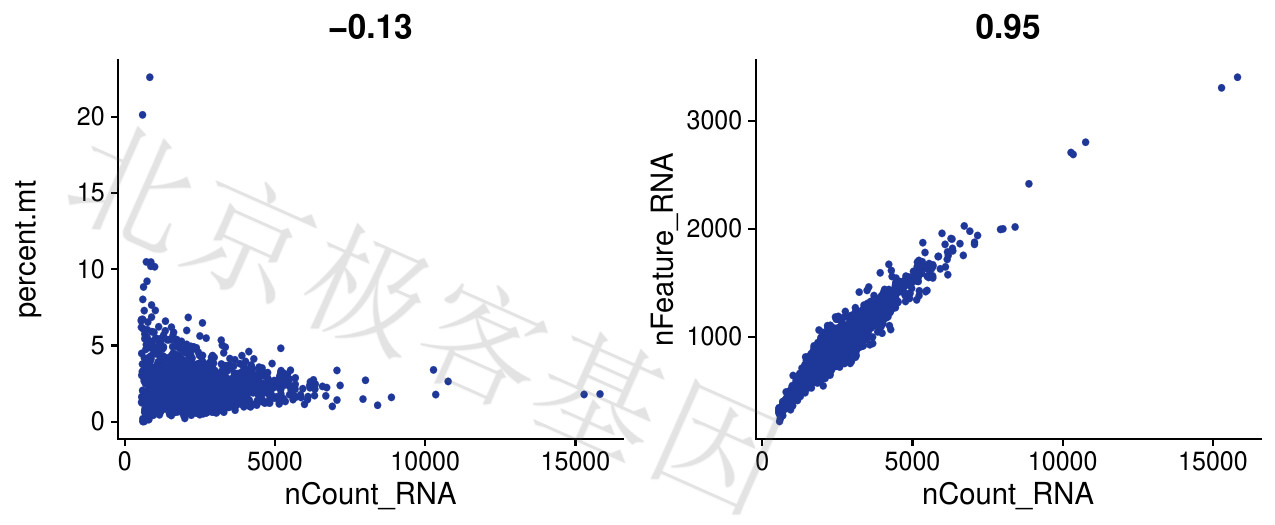
Figure 4.4: T-3个指标相互关系
PDF 文件 : T-feature_relationships.jpg
图例[Figure legends]
以nFeature_RNA和nCount_RNA之间的关系图为例,可以看到非常明显的一个相关性。假如nFeature_RNA个数为2000时对应的nCount_RNA在10000左右。那么设定阈值时,我们想过滤掉nFeature_RNA大于2000的细胞,此时umi的阈值就应该设置在10000左右。
Take the relationship diagram between nFeature_RNA and nCount_RNA as an example, you can see a very obvious correlation. If the number of nFeature_RNA is 2000, the corresponding nCount_RNA is around 10,000. Then when setting the threshold, we want to filter out cells with nFeature_RNA greater than 2000. At this time, the threshold of umi should be set at about 10,000.
4.3 过滤[Filter]
结合以上两种图表,将过滤参数设置为:nFeature_RNA去掉较低的0.1%,和较高的0.1%。
Combine the above two charts, set the filter parameters to: nFeature_RNA remove at least 0.1%, and higher 0.1%.